[A-00170]Apache Beamをローカルで動かしてみる
Apache Beamネタです。Google CloudのDataflowを触る上で、まずはBeamをSDKでローカル実行してみます。
・プロジェクトを作成する
今回はMaven+Java(JDK20)を使って作ってみます。
プロジェクト構成はMavenのsimple-architecureで作ってます。
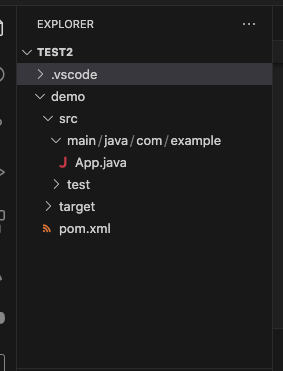
resourceディレクトリはありません。Javaクラスのみ格納できるディレクトリパスを作成してください。
・pom.xmlの定義
今回必要な依存関係はbeam-sdks-java-coreとbeamの実行に必要なbeam-runners-direct-javaになります。あとはslf4jとかを適当に入れておけば動くようになります。下記の例ではgoogleのapiなどが入ってますが、後々Dataflowで動かす際に必要になりますので気にしないでください。
<?xml version="1.0" encoding="UTF-8"?>
<project xmlns="http://maven.apache.org/POM/4.0.0" xmlns:xsi="http://www.w3.org/2001/XMLSchema-instance"
xsi:schemaLocation="http://maven.apache.org/POM/4.0.0 http://maven.apache.org/xsd/maven-4.0.0.xsd">
<modelVersion>4.0.0</modelVersion>
<groupId>com.example</groupId>
<artifactId>demo</artifactId>
<version>1.0-SNAPSHOT</version>
<name>demo</name>
<!-- FIXME change it to the project's website -->
<url>http://www.example.com</url>
<properties>
<beam.version>2.51.0</beam.version>
<bigquery.version>v2-rev20220924-2.0.0</bigquery.version>
<project.build.sourceEncoding>UTF-8</project.build.sourceEncoding>
<maven.compiler.source>20</maven.compiler.source>
<maven.compiler.target>20</maven.compiler.target>
<google-api-client.version>2.0.0</google-api-client.version>
<guava.version>32.0.1-jre</guava.version>
<pubsub.version>v1-rev20220904-2.0.0</pubsub.version>
<libraries-bom.version>26.17.0</libraries-bom.version>
</properties>
<dependencies>
<!-- Adds a dependency on the Beam Google Cloud Platform IO module. -->
<dependency>
<groupId>org.apache.beam</groupId>
<artifactId>beam-sdks-java-io-google-cloud-platform</artifactId>
<version>${beam.version}</version>
</dependency>
<!-- Dependencies below this line are specific dependencies needed by the examples code. -->
<dependency>
<groupId>com.google.api-client</groupId>
<artifactId>google-api-client</artifactId>
<version>${google-api-client.version}</version>
<exclusions>
<!-- Exclude an old version of guava that is being pulled
in by a transitive dependency of google-api-client -->
<exclusion>
<groupId>com.google.guava</groupId>
<artifactId>guava-jdk5</artifactId>
</exclusion>
</exclusions>
</dependency>
<dependency>
<groupId>com.google.apis</groupId>
<artifactId>google-api-services-bigquery</artifactId>
<version>${bigquery.version}</version>
<exclusions>
<!-- Exclude an old version of guava that is being pulled
in by a transitive dependency of google-api-client -->
<exclusion>
<groupId>com.google.guava</groupId>
<artifactId>guava-jdk5</artifactId>
</exclusion>
</exclusions>
</dependency>
<dependency>
<groupId>com.google.http-client</groupId>
<artifactId>google-http-client</artifactId>
<exclusions>
<!-- Exclude an old version of guava that is being pulled
in by a transitive dependency of google-api-client -->
<exclusion>
<groupId>com.google.guava</groupId>
<artifactId>guava-jdk5</artifactId>
</exclusion>
</exclusions>
</dependency>
<dependency>
<groupId>com.google.apis</groupId>
<artifactId>google-api-services-pubsub</artifactId>
<version>${pubsub.version}</version>
<exclusions>
<!-- Exclude an old version of guava that is being pulled
in by a transitive dependency of google-api-client -->
<exclusion>
<groupId>com.google.guava</groupId>
<artifactId>guava-jdk5</artifactId>
</exclusion>
</exclusions>
</dependency>
<!-- https://mvnrepository.com/artifact/org.apache.beam/beam-sdks-java-core -->
<dependency>
<groupId>org.apache.beam</groupId>
<artifactId>beam-sdks-java-core</artifactId>
<version>2.51.0</version>
</dependency>
<!-- https://mvnrepository.com/artifact/org.apache.beam/beam-runners-direct-java -->
<dependency>
<groupId>org.apache.beam</groupId>
<artifactId>beam-runners-direct-java</artifactId>
<version>2.51.0</version>
</dependency>
<dependency>
<groupId>org.slf4j</groupId>
<artifactId>slf4j-jdk14</artifactId>
<version>1.7.32</version>
</dependency>
<dependency>
<groupId>junit</groupId>
<artifactId>junit</artifactId>
<version>4.11</version>
<scope>test</scope>
</dependency>
</dependencies>
<dependencyManagement>
<dependencies>
<dependency>
<groupId>com.google.guava</groupId>
<artifactId>guava</artifactId>
<version>${guava.version}</version> <!-- "-jre" for Java 8 or higher -->
</dependency>
<!-- GCP libraries BOM sets the version for google http client -->
<dependency>
<groupId>com.google.cloud</groupId>
<artifactId>libraries-bom</artifactId>
<version>${libraries-bom.version}</version>
<type>pom</type>
<scope>import</scope>
</dependency>
</dependencies>
</dependencyManagement>
<build>
<pluginManagement><!-- lock down plugins versions to avoid using Maven defaults (may be moved to parent pom) -->
<plugins>
<!-- clean lifecycle, see https://maven.apache.org/ref/current/maven-core/lifecycles.html#clean_Lifecycle -->
<plugin>
<artifactId>maven-clean-plugin</artifactId>
<version>3.1.0</version>
</plugin>
<!-- default lifecycle, jar packaging: see https://maven.apache.org/ref/current/maven-core/default-bindings.html#Plugin_bindings_for_jar_packaging -->
<plugin>
<artifactId>maven-resources-plugin</artifactId>
<version>3.0.2</version>
</plugin>
<plugin>
<artifactId>maven-compiler-plugin</artifactId>
<version>3.8.0</version>
</plugin>
<plugin>
<artifactId>maven-surefire-plugin</artifactId>
<version>2.22.1</version>
</plugin>
<plugin>
<artifactId>maven-jar-plugin</artifactId>
<version>3.0.2</version>
</plugin>
<plugin>
<artifactId>maven-install-plugin</artifactId>
<version>2.5.2</version>
</plugin>
<plugin>
<artifactId>maven-deploy-plugin</artifactId>
<version>2.8.2</version>
</plugin>
<!-- site lifecycle, see https://maven.apache.org/ref/current/maven-core/lifecycles.html#site_Lifecycle -->
<plugin>
<artifactId>maven-site-plugin</artifactId>
<version>3.7.1</version>
</plugin>
<plugin>
<artifactId>maven-project-info-reports-plugin</artifactId>
<version>3.0.0</version>
</plugin>
</plugins>
</pluginManagement>
</build>
<profiles>
<profile>
<id>dataflow-runner</id>
<!-- Makes the DataflowRunner available when running a pipeline. -->
<dependencies>
<dependency>
<groupId>org.apache.beam</groupId>
<artifactId>beam-runners-google-cloud-dataflow-java</artifactId>
<version>${beam.version}</version>
<scope>runtime</scope>
</dependency>
</dependencies>
</profile>
</profiles>
</project>
次に実際に動くコードは下記のApp.javaです。
package com.example;
import java.util.Arrays;
import org.apache.beam.sdk.Pipeline;
import org.apache.beam.sdk.options.Default;
import org.apache.beam.sdk.options.Description;
import org.apache.beam.sdk.options.PipelineOptionsFactory;
import org.apache.beam.sdk.options.StreamingOptions;
import org.apache.beam.sdk.transforms.Create;
import org.apache.beam.sdk.transforms.MapElements;
import org.apache.beam.sdk.values.PCollection;
import org.apache.beam.sdk.values.TypeDescriptors;
/**
* Hello world!
*
*/
public class App {
public interface Options extends StreamingOptions {
@Description("Input text to print.")
@Default.String("My input text")
String getInputText();
void setInputText(String value);
}
public static PCollection<String> buildPipeline(Pipeline pipeline, String inputText) {
return pipeline.apply("Create elements", Create.of(Arrays.asList("Hello", "World!", inputText)))
.apply("Print elements"
, MapElements.into(TypeDescriptors.strings()).via(x -> {
System.out.println(x);
return x;
}));
}
public static void main(String[] args) {
var options = PipelineOptionsFactory.fromArgs(args).withValidation().as(Options.class);
var pipeline = Pipeline.create(options);
App.buildPipeline(pipeline, options.getInputText());
pipeline.run().waitUntilFinish();
}
}
簡単なHello,worldプログラムになっています。上記を作成したら、mvnコマンドでclean,installを実行します。
ビルドが完了したら下記のコマンドをターミナルから実行してプログラムを動かしてみます。
mvn compile exec:java \
-Dexec.mainClass=com.example.App \
-Dexec.args="--inputText=Wow"
上記を実行すると下記のようにログが出力されます。
(base) MacBook-Pro:demo$ mvn compile exec:java \
> -Dexec.mainClass=com.example.App \
> -Dexec.args="--inputText=Wow"
[INFO] Scanning for projects...
[INFO]
[INFO] --------------------------< com.example:demo >--------------------------
[INFO] Building demo 1.0-SNAPSHOT
[INFO] from pom.xml
[INFO] --------------------------------[ jar ]---------------------------------
[INFO]
[INFO] --- resources:3.0.2:resources (default-resources) @ demo ---
[INFO] Using 'UTF-8' encoding to copy filtered resources.
[INFO] skip non existing resourceDirectory /Users/anonymous/Documents/practice/apache-beam/test2/demo/src/main/resources
[INFO]
[INFO] --- compiler:3.8.0:compile (default-compile) @ demo ---
[INFO] Nothing to compile - all classes are up to date
[INFO]
[INFO] --- exec:3.1.0:java (default-cli) @ demo ---
Oct 31, 2023 2:10:18 AM org.apache.beam.repackaged.direct_java.runners.core.construction.Environments$JavaVersion forSpecification
WARNING: unsupported Java version: 20, falling back to: 17
Wow
World!
Hello
[INFO] ------------------------------------------------------------------------
[INFO] BUILD SUCCESS
[INFO] ------------------------------------------------------------------------
[INFO] Total time: 8.531 s
[INFO] Finished at: 2023-10-31T02:10:19+09:00
[INFO] ------------------------------------------------------------------------
Java20はサポートしてないという警告が出ますがとりあえず動きますのでよしとします。
以上がApache Beamをローカルで動かす方法でした。
・WordCountパイプラインを作成する
ワードの数をカウントするパイプラインです。GCS上に置いたファイルをカウントします。
下記のコマンドでテキストファイルをダウンロードできます。
gsutil cp gs://apache-beam-samples/shakespeare/kinglear.txt ./
下記のプログラムを実行します。
package com.example.etl.beam;
import java.util.Arrays;
import org.apache.beam.sdk.Pipeline;
import org.apache.beam.sdk.io.TextIO;
import org.apache.beam.sdk.options.PipelineOptions;
import org.apache.beam.sdk.options.PipelineOptionsFactory;
import org.apache.beam.sdk.transforms.Count;
import org.apache.beam.sdk.transforms.Filter;
import org.apache.beam.sdk.transforms.FlatMapElements;
import org.apache.beam.sdk.transforms.MapElements;
import org.apache.beam.sdk.values.KV;
import org.apache.beam.sdk.values.TypeDescriptors;
public class GcsTextReadPipeline {
public static void main(String[] args) {
PipelineOptions options = PipelineOptionsFactory.create();
Pipeline p = Pipeline.create(options);
p.apply(TextIO.read().from("gs://xxxx/kinglear.txt"))
.apply(FlatMapElements.into(TypeDescriptors.strings()).via(
(String line) -> Arrays.asList(line.split("[^\\p{L}]+"))
))
.apply(
Filter.by(
(String word) -> !word.isEmpty()
))
.apply(Count.perElement())
.apply(
MapElements.into(TypeDescriptors.strings())
.via(
(KV<String, Long> wordCount) -> wordCount.getKey() + ": " + wordCount.getValue()
))
.apply(TextIO.write().to("wordcounts"));
p.run().waitUntilFinish();
}
}
mvn compile exec:java -Dexec.mainClass=com.example.etl.beam.GcsTextReadPipeline
実行すると下記のようにファイルを作成します。
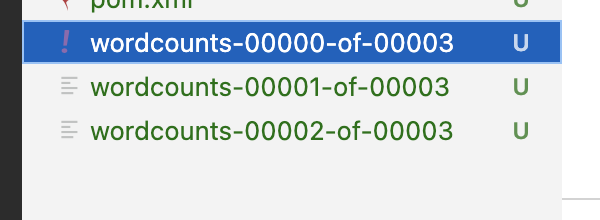
・Partitionを使ってみる
特定のグループでパーティションを組むBeamの関数を使った例です。
package com.example.etl.beam;
import org.apache.beam.sdk.Pipeline;
import org.apache.beam.sdk.transforms.Create;
import org.apache.beam.sdk.transforms.DoFn;
import org.apache.beam.sdk.transforms.ParDo;
import org.apache.beam.sdk.transforms.Partition;
import org.apache.beam.sdk.values.PCollection;
import org.apache.beam.sdk.values.PCollectionList;
public class PartitionPipeline {
public static void main(String[] args) {
Pipeline p = Pipeline.create();
PCollection<Double> reviews = p.apply(Create.of(4.5,3.8,5.0,4.2,3.6));
int numPartitions = 2;
PCollectionList<Double> partitionReviews = reviews.apply(
Partition.of(numPartitions, new Partition.PartitionFn<Double>() {
public int partitionFor(Double elem, int numPartitions) {
return (elem >= 4.0) ? 0 : 1;
}
}));
partitionReviews.get(0).apply("PrintPositiveReviews", ParDo.of(new PrintFn("Positive Review")));
partitionReviews.get(1).apply("PrintNegativeReviews", ParDo.of(new PrintFn("Negative Review")));
p.run().waitUntilFinish();
}
static class PrintFn extends DoFn<Double, Void> {
private String type;
PrintFn(String type) {
this.type = type;
}
@ProcessElement
public void processElement(@Element Double review) {
System.out.println(type + ": " + review);
}
}
}
mvn compile exec:java -Dexec.mainClass=com.example.etl.beam.PartitionPipeline
Negative Review: 3.6
Positive Review: 4.2
Positive Review: 5.0
Positive Review: 4.5
Negative Review: 3.8
・FlatMapElementsにワードを分解して要素を詰める
文章を分解してどのようなワードが現れたかを要素分解するプログラムです。FlatMapElementsのサンプルです。
package com.example.etl.beam;
import java.util.Arrays;
import org.apache.beam.sdk.Pipeline;
import org.apache.beam.sdk.transforms.Create;
import org.apache.beam.sdk.transforms.DoFn;
import org.apache.beam.sdk.transforms.FlatMapElements;
import org.apache.beam.sdk.transforms.ParDo;
import org.apache.beam.sdk.values.PCollection;
import org.apache.beam.sdk.values.TypeDescriptors;
public class WordExtractPipeline {
public static void main(String[] args) {
Pipeline p = Pipeline.create();
PCollection<String> reviews = p.apply(Create.of(Arrays.asList("Thank you Neighbor", "Works well for the price")));
PCollection<String> words = reviews.apply(
FlatMapElements.into(TypeDescriptors.strings())
.via((review) -> Arrays.asList(review.split(" ")))
);
words.apply(ParDo.of(new DoFn<String,Void>() {
@ProcessElement
public void processElement(@Element String value) {
System.out.println(value);
}
}));
p.run().waitUntilFinish();
}
}
mvn compile exec:java -Dexec.mainClass=com.example.etl.beam.WordExtractPipeline
Works
Thank
well
for
you
the
Neighbor
price
・リスト要素をマージするFlatten
Flatten関数の使い方サンプルです。Listの要素をマージします。マージ後の順序はランダムのようです。
package com.example.etl.beam;
import org.apache.beam.sdk.Pipeline;
import org.apache.beam.sdk.transforms.Create;
import org.apache.beam.sdk.transforms.DoFn;
import org.apache.beam.sdk.transforms.Flatten;
import org.apache.beam.sdk.transforms.ParDo;
import org.apache.beam.sdk.values.PCollection;
import org.apache.beam.sdk.values.PCollectionList;
public class ProductReviewMergePipeline {
public static void main(String[] args) {
Pipeline p = Pipeline.create();
PCollection<String> review1 = p.apply(Create.of("Excellent", "Very good"));
PCollection<String> review2 = p.apply(Create.of("Average", "Good"));
PCollection<String> mergedReviews = PCollectionList.of(review1).and(review2)
.apply(Flatten.pCollections());
mergedReviews.apply(ParDo.of(new DoFn<String,Void>() {
@ProcessElement
public void processElement(@Element String review) {
System.out.println(review);
}
}));
p.run().waitUntilFinish();
}
}
mvn compile exec:java -Dexec.mainClass=com.example.etl.beam.ProductReviewMergePipeline
Excellent
Average
Good
Very good
・KEYで要素をグループ化するGroupByKey
MapエレメントのKEYを元にグループ化するGroupByKey関数のサンプルです。
package com.example.etl.beam;
import org.apache.beam.sdk.Pipeline;
import org.apache.beam.sdk.transforms.Create;
import org.apache.beam.sdk.transforms.DoFn;
import org.apache.beam.sdk.transforms.GroupByKey;
import org.apache.beam.sdk.transforms.ParDo;
import org.apache.beam.sdk.values.KV;
import org.apache.beam.sdk.values.PCollection;
public class GroupByKeyPipeline {
public static void main(String[] args) {
Pipeline p = Pipeline.create();
PCollection<KV<String, String>> makers = p.apply(Create.of(KV.of("Electric Guitar Makers", "Fender"),
KV.of("Electric Guitar Makers", "Gibson"),
KV.of("Saxophone Makers", "Selmer"),
KV.of("Saxophone Makers", "Buffet Crampon")));
PCollection<KV<String, Iterable<String>>> aggregatedMakers = makers.apply(GroupByKey.create());
aggregatedMakers.apply(ParDo.of(new DoFn< KV<String, Iterable<String>>, Void>() {
@ProcessElement
public void processElement(@Element KV<String, Iterable<String>> kv ) {
System.out.println(kv.getKey() + " : " + kv.getValue());
}
}));
p.run().waitUntilFinish();
}
}
mvn compile exec:java -Dexec.mainClass=com.example.etl.beam.GroupByKeyPipeline
Saxophone Makers : [Buffet Crampon, Selmer]
Electric Guitar Makers : [Gibson, Fender]
・特定の要素のみに絞るFilter
Filter関数を使えば特定の要素のみに絞ることが可能です。
package com.example.etl.beam;
import org.apache.beam.sdk.Pipeline;
import org.apache.beam.sdk.transforms.Create;
import org.apache.beam.sdk.transforms.DoFn;
import org.apache.beam.sdk.transforms.Filter;
import org.apache.beam.sdk.transforms.ParDo;
public class FilterablePipeline {
public static void main(String[] args) {
Pipeline p = Pipeline.create();
p.apply(Create.of("This product is absolutely amazing.", "It's okay, but I've seen better.",
"Fantastic quality and design.", "I didn't like this product at all."))
.apply(Filter.by(review -> review.contains("amazing") || review.contains("Fantastic")))
.apply(ParDo.of(new PrintReviewFn()));
p.run().waitUntilFinish();
}
static class PrintReviewFn extends DoFn<String, Void> {
@ProcessElement
public void processElement(@Element String review) {
System.out.println("Positive Review : " + review);
}
}
}
mvn compile exec:java -Dexec.mainClass=com.example.etl.beam.FilterablePipeline
Positive Review : Fantastic quality and design.
Positive Review : This product is absolutely amazing.
・平均値を求めるMean.globally
Mean.globallyを使えば平均値が求められます。
package com.example.etl.beam;
import org.apache.beam.sdk.Pipeline;
import org.apache.beam.sdk.transforms.Create;
import org.apache.beam.sdk.transforms.DoFn;
import org.apache.beam.sdk.transforms.Mean;
import org.apache.beam.sdk.transforms.ParDo;
import org.apache.beam.sdk.values.PCollection;
public class ComputeAverageRatingPipeline {
public static void main(String[] args) {
Pipeline p = Pipeline.create();
PCollection<Double> productRatings = p.apply(Create.of(4.5, 3.8, 5.0,4.2, 3.6 ));
PCollection<Double> avgRatings = productRatings.apply("Compute Average Ratings", Mean.globally());
avgRatings.apply(ParDo.of(new PrintFn()));
p.run().waitUntilFinish();
}
static class PrintFn extends DoFn<Double, Void> {
@ProcessElement
public void processElement(@Element Double avgRating ) {
System.out.println("Avg Rating is : " + avgRating);
}
}
}
mvn compile exec:java -Dexec.mainClass=com.example.etl.beam.ComputeAverageRatingPipeline
Avg Rating is : 4.22
・データベース連携してみる
とりあえずbigtable, bigquery, spannerという三つのデータベースとcloud pub/subに繋いでローカルでbeamを実行してみたいと思います。
bigtableとspannerは課金が高いのでemulatorを使用します。
・Appendix
公式文献はこちら
https://cloud.google.com/dataflow/docs/guides/deploying-a-pipeline?hl=ja
https://cloud.google.com/dataflow/docs/quickstarts/create-pipeline-java?hl=ja
https://beam.apache.org/documentation/basics
参考文献はこちら
https://stackoverflow.com/questions/69771678/how-to-add-apache-beam-direct-runner-to-classpath
https://zenn.dev/hashito/articles/f036b50c3c33d1
https://www.baeldung.com/jackson-deserialize-json-unknown-properties
「[A-00170]Apache Beamをローカルで動かしてみる」への0件のコメント